In-Depth Look at Schwab's Algorithmic Trading Strategies
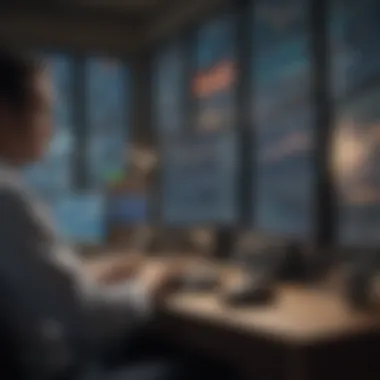
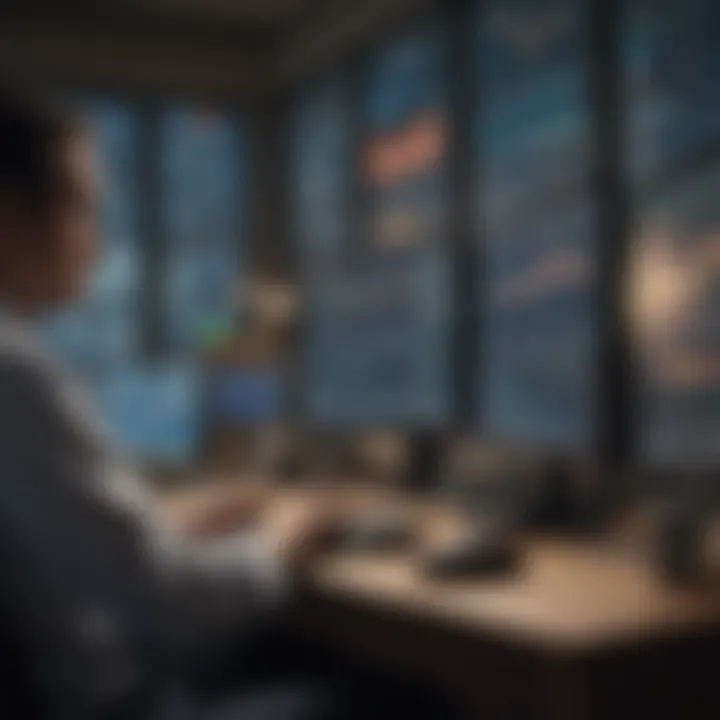
Intro
In today's fast-paced financial arena, the intersection of technology and investment has become a significant player. No longer are investors solely relying on gut feelings or traditional means of trading. Instead, automated systems, particularly through Schwab's algorithmic trading, have ushered in a new era. This narrative aims to unravel the complexities behind these trading strategies, offering insights that span from their fundamental workings to their broader implications for both amateur and seasoned investors.
Algorithmic trading, in its simplest essence, refers to the use of computer programs to execute trades at high speeds. These algorithms can analyze vast amounts of data, allowing traders to leverage even the minutest market movements. However, as simple as it sounds, the reality is layered with intricate details that necessitate a deeper understanding.
By exploring Schwab's specific approach, we gain valuable perspectives on how these strategies are crafted, their benefits, and the inherent risks involved. In an environment where the stakes are always high, knowing how to navigate these waters is crucial for anyone looking to optimize their trading activities.
Understanding Algorithmic Trading
Algorithmic trading has reshaped the financial markets in multiple ways. It serves as the backbone of most modern trading strategies, orchestrating trades faster than any human could manage, while also providing insightful market analysis. For both novice and seasoned investors, grasping the fundamentals of algorithmic trading is essential. This section will outline its definition, significance, and the ramifications of its evolution over the years.
Definition and Fundamentals
To comprehend algorithmic trading, itâs crucial to start with a solid definition. At its core, algorithmic trading refers to the use of computer algorithms to execute trades based on predefined criteria. These algorithms can assess numerous variablesâmarket data, price movements, historical trendsâand make split-second decisions which can provide a competitive edge over traditional methods.
Benefits of algorithmic trading include:
- Speed: Trades are executed in milliseconds. Manual entries, naturally, take considerably longer.
- Accuracy: By removing human emotion from trading, algorithms minimize the risk of mistakes due to psychological factors.
- Backtesting: Investors can test strategies on historical data before implementation, thereby refining tactics without exposing themselves to actual market risk.
- Consistency: Algorithmic trading ensures adherence to a strategy without straying due to emotionsâall parameters are strictly followed.
Historical Context of Algorithmic Trading
Understanding the historical evolution of algorithmic trading underscores its significance today. The roots of algorithmic trading can be traced back to the 1970s when stock exchanges began adopting electronic trading systems. However, it wasn't until the late 1990s that algorithmic trading burgeoned, largely thanks to advances in technology and data processing capabilities.
This phase also saw more sophisticated algorithms developed, capable of trading on multiple markets simultaneously. As technology has advanced, so have the algorithmsâ moving from basic execution strategies to complex models that can analyze vast data sets in real-time. This has led to their widespread adoption, often accounting for over 70% of trading volume in some markets.
"The marriage of technology and finance has created a new order of tradingâone that no longer relies solely on human intuition but is driven by numeric strategy and complex patterns."
Today, algorithmic trading isn't confined to institutional investors; even retail traders can leverage these tools via platforms such as Schwab's. However, navigating this landscape requires an understanding not just of how algorithms work, but also of the inherent risks involved. As we explore Schwab's role, it'll be imperative to keep these fundamental elements in mind.
Schwab's Role in Algorithmic Trading
The role that Schwab plays in the realm of algorithmic trading is one that intertwines innovation with the practicality demanded by investors. As an established brokerage firm, Schwab has carved out a significant niche in a fast-paced trading landscape, offering both novices and seasoned traders access to sophisticated trading tools. Itâs not just about having the broadest array of products; itâs about enhancing the trading experience through technology.
In this section, we examine how Schwab integrates algorithmic trading into its offerings. Understanding their approach gives insight into their commitment to facilitating more informed, data-driven decision-making processes for their clients.
Overview of Schwab's Trading Platform
Schwabâs trading platform stands out due to its user-friendly interface and robust features. The platform is designed to accommodate a broad spectrum of trading needs, whether you're dipping your toes into the markets for the first time or you're a battle-hardened pro navigating complex strategies. Schwab has equipped its platform with various tools that range from basic stock trading to more intricate options, ETFs, and future trades.
Some key features include:
- Advanced Charting Tools: Traders can access real-time data, adjustable time frames, and a slew of technical indicators to fine-tune their trading approach.
- Market Analysis and Insights: Schwab provides in-depth market research baked right into their platform, giving traders the information they need at their fingertips.
- Mobile Trading Options: With the rise of mobile technology, Schwab ensures that users can trade from virtually anywhere, making it easy to seize opportunities as they arise.
The seamless integration of these features serves to establish Schwab not just as a trading platform but as a holistic trading ecosystem that supports the user's journey in the stock market.
Integration of Algorithms in Schwab's Services
Incorporating algorithms into Schwabâs suite of services has brought forth a new level of efficiency and precision in trading. By lowering the barrier to entry for algorithmic trading, Schwab enables individual investors to utilize sophisticated strategies that might have previously been only accessible to institutional players.
Here, it's worth noting some significant aspects of this integration:
- Automated Trading Strategies: Schwab allows clients to set parameters that enable the execution of trades based on market conditions, thus minimizing the emotional rollercoaster that often comes with investing.
- Personalized Algorithm Development: Clients can customize algorithms to suit their trading strategies or risk tolerance, making trading not just more accessible but also tailored.
- Integration with Educational Resources: Schwab doesn't just throw clients into the deep end; they offer comprehensive tools like webinars and tutorials, helping users understand the principles of algorithmic trading and its practical applications.
In this era of information overload, Schwab cuts through the noise, paving a pathway for investors to harness the power of algorithms in their trading strategies better.
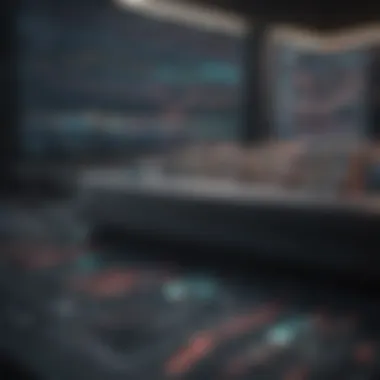
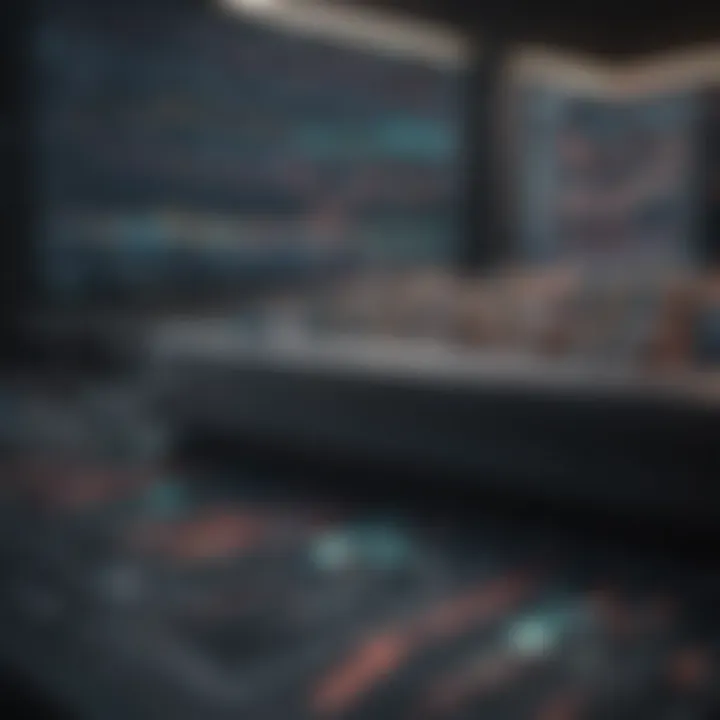
In summary, Schwabâs role in algorithmic trading rests not only on providing a robust platform and algorithms but also on empowering users through education and support. This combination fosters a more confident trading environment and a better grasp of complex market dynamics.
By weaving together technology, education, and user-centric services, Schwab positions itself as a vital ally for those looking to navigate the algorithmic trading landscape.
Mechanics of Schwab's Algorithms
Understanding the mechanics behind Schwab's algorithms is crucial for grasping how they function in todayâs fast-paced financial markets. Schwab's algorithmic trading leverages advanced mathematical and statistical models to make trading decisions more efficiently. This technology transforms how trades are executed, enhancing execution speed and accuracy.
Algorithm Types Employed by Schwab
Schwab employs a variety of algorithm types that cater to different trading strategies. These algorithms can be broadly categorized into several types:
- Execution Algorithms: These are designed to optimize the buying and selling process. They focus on minimizing costs associated with trading, particularly slippage, which can erode gains.
- Liquidity-Seeking Algorithms: These aim to find the best price in the market while taking into account the liquidity available. They can adjust their trading strategy based on market conditions, ensuring trades are filled without causing drastic price movements.
- Tactical Trading Algorithms: These are used for more complex strategies, including statistical arbitrage and pairs trading. They analyze historical data to identify potential trading opportunities that are not immediately apparent.
The choice of algorithm impacts not only the trade execution but also the overall success of the trading strategy. By employing such specialized algorithms, Schwab helps traders make informed decisions, potentially leading to better financial outcomes.
Data Processing and Real-Time Analysis
In today's trading environment, the ability to analyze data in real-time is paramount. Schwabâs algorithms utilize vast amounts of market dataâfrom price feeds to news releasesâto inform trading decisions almost instantaneously. This constant stream of data feeds into Schwabâs algorithms, allowing them to:
- React to Market Movements: Algorithms can process data much faster than a human could ever hope to, which is vital in volatile markets. For instance, a sudden shift in stock prices can prompt adjustments to trading strategies within fractions of a second.
- Leverage Machine Learning: Some of Schwab's models are based on machine learning, allowing them to adapt over time. This means algorithms can learn from past trades and outcomes, improving their predictions and recommendations.
The importance of data processing cannot be overstated; it allows Schwabâs traders to follow a systematic approach while minimizing human error. The insights gleaned from this data create a competitive edge, enabling traders to move swiftly and decisively in their strategies.
Real-time data analysis ensures that opportunities are not just speculated upon but acted on immediately, keeping Schwabâs platform at the forefront of algorithmic trading innovation.
Advantages of Algorithmic Trading
Algorithmic trading has become a cornerstone of modern financial markets. It's not just about speed; it's an intricate dance of technology and strategy that can redefine how investors, both novices and veterans alike, approach trading. This section lays bare the significance of algorithmic trading advantages while also addressing essential elements that could influence trading decisions.
Increased Efficiency and Speed
In the bustling world of finance, every millisecond counts. Algorithms inherently process large quantities of data at breakneck speeds. For instance, while a human trader might take time to digest market news or analyze trends, an algorithm could execute trades in a fraction of a second. This level of speed ensures that traders aren't just reacting to the market, but instead, leveraging patterns that they might not even consciously observe.
- Execution Precision: Algorithms are designed to optimize trade execution. By assessing various parametersâsuch as market depth, liquidity, and volatilityâthey can find the best possible entry and exit points. This level of precision can significantly reduce slippage, resulting in better prices for traders.
- Resource Management: Automated systems allow for optimal resource allocation. Imagine a scenario where a team of analysts needs to monitor multiple markets at once. With algorithmic trading, itâs all handled by powerful computers that can execute strategies without the fatigue humans might experience. This leads to better decision-making over the long haul.
However, embracing this kind of trading does necessitate a profound understanding of the underlying algorithms. Traders must ensure that they choose strategies aligned with their investment goals and risk tolerance.
Enhanced Market Analysis Capabilities
Algorithmic trading isnât just about executing orders; itâs about making sense of the market at a deeper level. The analytical capabilities of algorithms can offer insights that might otherwise go unnoticed.
- Data Processing Power: Algorithms can analyze real-time data where human analysts might falter. For instance, they can sift through economic indicators, earnings reports, and sentiment analysis to inform trading decisions. This means that traders can anticipate market movements based on comprehensive data reviews rather than limited perspectives.
- Pattern Recognition: Algorithms are particularly adept at identifying patterns in historical data. They can track price movements and trading volumes, looking for anomalies or high-probability setups for future trades. This is invaluable for both short-term trading strategies and long-term investment philosophies.
"In a world swamped with information, algorithms stand as beacons of clarity, guiding traders through the noise."
Adopting these advanced analytical tools can shift a trader's perspective, enabling them to make informed, strategic decisions rather than relying solely on instinct. The analytics provided by algorithmic trading can help investors adapt quicklyâeven if market conditions change in the blink of an eye.
Challenges and Risks
Exploring the world of Schwab's algorithmic trading brings to light numerous challenges and risks that investors and traders must consider. Algorithmic trading is not a silver bullet that guarantees profits; rather, it comes along with its own set of complexities. Recognizing these challenges can markedly enhance an investor's ability to make informed decisions in the fast-paced trading landscape. These potential pitfalls can lead to significant financial losses if administrators and traders do not take proper precautions.
Technical Failures and Glitches
Technical issues can strike when least expected, especially for platforms running complex algorithms. These failures can manifest in numerous ways: server outages, connectivity issues, or software bugs, just to name a few. When these glitches occur, they can disrupt trading operations, and thus affect an investorâs ability to buy or sell assets at the opportune moment.
For instance, a trader relying on Schwabâs algorithms to execute trades might find themselves at a loss due to a sudden system crash. Such a scenario translates into missed opportunities and potentially substantial financial setbacks.
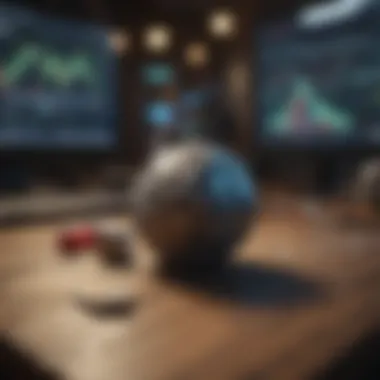
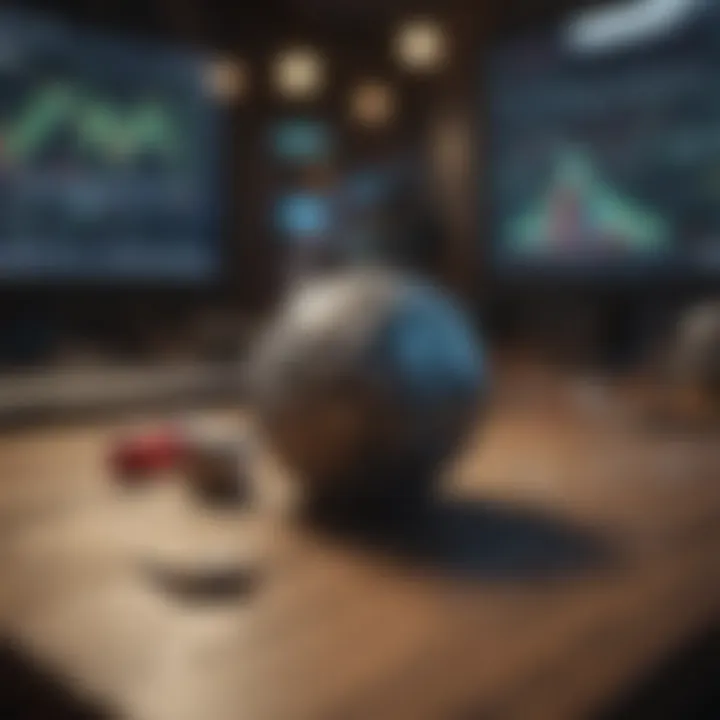
- Potential Causes of Failures:
- Impact on Traders:
- Outdated technology
- Network disruptions
- Software bugs or errors
- Inability to execute trades
- Delayed response times
- Escalating slippage (the difference between expected and executed price)
Technical failures underscore the importance of having robust systems in place, along with contingency plans that traders can activate to minimize damage. Spearheading continuous monitoring and updates can often help keep such issues at bay.
Market Manipulation Concerns
Algorithmic trading, while efficient, runs the risk of exacerbating market manipulation concerns. As algorithms act on predefined rules, they can create such rapid price movements that they attract undue attention. This may lead to situations where less scrupulous market players could potentially manipulate prices to their advantage.
Market manipulation comes in various forms, from spoofingâwhere a trader places orders they have no intention of executingâto layering, where traders create a flurry of submissions to confuse the market. These behaviors can distort market prices, misguiding algos into unfavorable trading decisions.
"Understanding the risks related to market manipulation is crucial for the sustainibility of algorithm-based trading."
Addressing these concerns calls for stringent adherence to regulations. Algorithmic traders must stay informed about legal standards to mitigate the likelihood of inadvertently crossing ethical lines. A proactive approach towards compliance, alongside diligent monitoring of market activities, can prove invaluable in holding manipulation at bay.
In summary, navigating the challenges of algorithmic trading on Schwab's platform requires keen attention to both technical pitfalls and market integrity. By understanding potential failing points and the risks of market manipulation, traders can foster a more strategic and secure trading approach.
Risk Management Techniques
Risk management in algorithmic trading is akin to sailing a ship through tumultuous waters. Itâs not about avoiding storms but rather navigating through them with a sturdy hull and a skilled crew. This section explores the importance of risk management techniques in Schwab's algorithmic trading strategies, emphasizing how they can save traders from the choppy seas of financial markets.
When trading algorithms operate, markets can be unpredictable. Prices can swing wildly in a matter of seconds, influenced by a myriad of factors like economic reports or geopolitical tensions. Having robust risk management strategies in place is crucial for both novice and seasoned traders. These techniques help in safeguarding investments, minimizing losses, and ultimately enhancing the potential for profitable trades.
Setting Precise Parameters
Setting precise parameters is the first line of defense in algorithmic trading. This involves establishing clear rules for the algorithm to follow, which govern how it reacts under various market conditions. When investors dictate precise entry and exit points, they effectively diminish the likelihood of making impulsive decisions driven by market emotions.
For instance, an algorithm set to buy a specific stock when it dips below a predetermined price helps traders capitalize on bargains. Likewise, specifying the threshold for selling a stock mitigates loss by preventing further declines in value. More than just numbers, these parameters set a discipline that guides trading behaviors.
Among the vital aspects of setting parameters are:
- Risk Tolerance: Understand how much loss is acceptable before executing trades.
- Market Conditions: Define how the algorithm should behave in different market scenariosâfor example, being more conservative during high volatility.
- Strategy Optimization: Periodically reassess and fine-tune parameters based on past performance data.
Stop-Loss and Take-Profit Orders
Stop-loss and take-profit orders are essentially the safety nets that every algorithmic trader should have in place. These orders act as automatic triggers to either cut losses or lock in profits, reducing the emotional burden of trading.
A stop-loss order automatically sells an asset when it hits a predefined price. This helps protect against unforeseen downturns. Conversely, a take-profit order secures gains by selling an asset when it reaches a certain profit level. Implementation of these orders provides peace of mind, knowing that the trade will behave in a predetermined manner based on market movements.
Incorporating stop-loss and take-profit orders involves understanding the following details:
- Market Analysis: Examine historical data to determine the best levels for these orders.
- Dynamic Adjustments: Traders can tweak orders as conditions change, especially in fast-moving markets.
- Psychological Impact: These orders can help mitigate the emotional toll of watching a trade fluctuate, as they automate decisions based on rational parameters rather than panic or greed.
"Effective risk management ensures that traders can weather the inevitable ups and downs of the market, preserving capital and seizing opportunities when they arise."
Real-World Applications
The significance of real-world applications of algorithmic trading cannot be overstated, especially in the context of Schwab's platform. As more investors seek to maximize their returns, the necessity for effective trading strategies that yield measurable results becomes ever more pressing. Schwabâs algorithmic trading capabilities provide valuable tools that meet this demand, serving both novice and experienced traders.
The practical implications of algorithmic trading can be summed up in a few key benefits:
- Efficiency: Automated trading algorithms execute trades significantly quicker than manual methods. This speed can be critical in fast-moving markets where delays could lead to missed opportunities.
- Precision: Algorithms can analyze vast amounts of data without the emotional biases that often affect human decision-making. This precision aids investors in making well-informed choices based on statistical analysis rather than intuition.
- Access to Complex Strategies: Many average investors wouldnât have the expertise to develop intricate trading strategies from scratch. Schwabâs platform simplifies this through algorithmic solutions that even beginners can deploy after a little bit of learning.
- Risk Diversification: With algorithmic trading, investors can simultaneously manage multiple trades across different assets, spreading risk while optimizing potential returns.
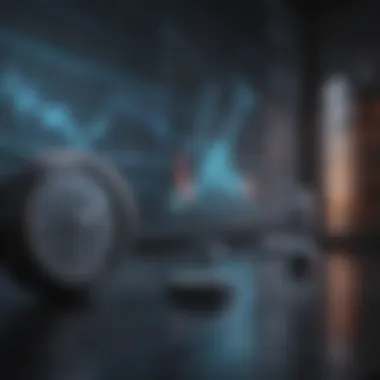
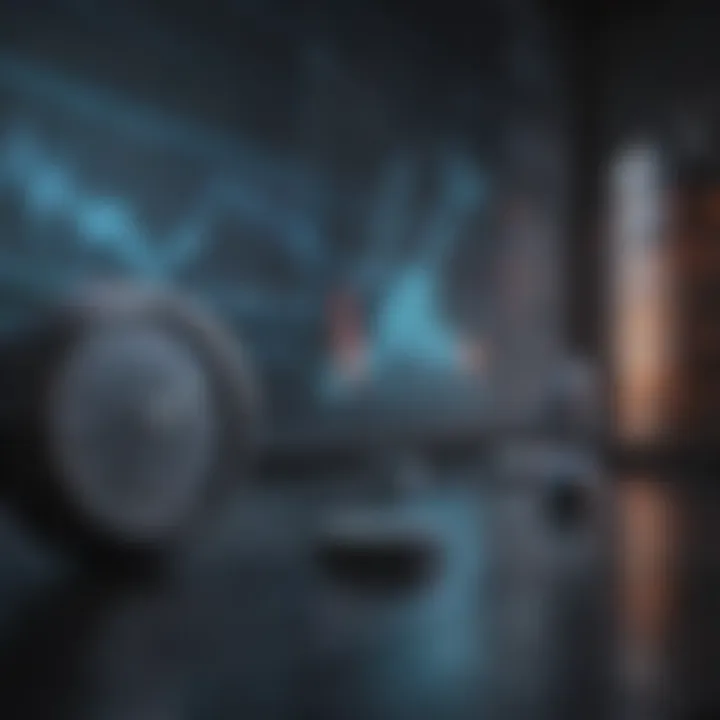
Case Studies of Successful Trades
To illustrate how Schwab's algorithmic trading can lead to successful outcomes, several case studies stand out. For instance, a retail investor using Schwabâs algorithm was able to achieve a 20% return in a single fiscal quarter by leveraging the platform's automated trend identification features. These algorithms identified a rising trend in technology stocks due to increased consumer demand amid changing market conditions.
These algorithms process various signals, quickly spotting patterns that might escape the naked eye. The investor received alerts on strategic entry and exit points, allowing them to capitalize on the favorable conditions without constantly monitoring the market.
Additionally, another case involved an institutional investor utilizing Schwab's algorithm for high-frequency trading. The algorithm executed hundreds of trades per minute, successfully capitalizing on micro-fluctuations in stock prices. In a situation where human input simply wouldn't keep pace, the algorithm's efficiency led to profits that would not have been possible otherwise.
Feedback from Users on Schwab's Platform
User feedback provides another lens to examine the effectiveness of Schwab's algorithmic trading. Many users note an appreciation for the intuitiveness of the platform, particularly when setting up automated strategies.
For example, a seasoned investor remarked, "Schwabâs algorithm tools have transformed my approach. I can now set parameters and let the system do the heavy lifting."
However, it's worth mentioning that some users have expressed the need for more educational resources specific to algorithmic trading. A recurring theme in feedback is that while the platform is user-friendly, a segment of novice investors feel overwhelmed by the choices they must make when configuring their algorithms. They suggest an increase in tutorial content or webinars focused on practical applications and best practices.
The Regulatory Environment
The world of algorithmic trading doesn't operate in a vacuum. It's tightly woven into a fabric of regulations designed to maintain market integrity and protect investors. This regulatory environment is critical for various reasons. It helps ensure that algorithmic trading practices do not contribute to market manipulation or systemic risks. Additionally, regulations provide a framework that can lead to more transparency and accountability, which are essential in todayâs fast-paced trading landscape. Understanding these regulations is not just for compliance; it sets the stage for risk management and strategic decision-making.
Overview of Applicable Regulations
In the realm of algorithmic trading, there are several key regulations that traders must navigate. Institutions such as the Securities and Exchange Commission (SEC) and the Commodity Futures Trading Commission (CFTC) have established overarching rules that govern trading practices.
Some essential regulations include:
- Regulation National Market System (NMS): This set of rules ensures fair access to markets and requires that trades occur at the best available price.
- Market Access Rule: This rule requires broker-dealers to have controls in place to prevent erroneous trades, such as those caused by faulty algorithms.
- MiFID II (Markets in Financial Instruments Directive II): Primarily applicable in Europe, this regulation compels firms to be transparent about trading and puts limits on high-frequency trading practices.
- Dodd-Frank Act: This act imposed regulations on derivatives trading and overseen the implementation of risk management practices.
Understanding these regulations assists traders in not only staying compliant but also optimizing their trading strategies. Being acquainted with these rules allows for a more strategic approach, potentially reducing the risks tied to algorithmic trading.
Compliance Challenges for Algorithmic Traders
Navigating the regulatory landscape can feel like walking through a minefieldâone wrong step and you might set off alarms. Compliance with regulations is not as straightforward as it seems. Several challenges confront algorithmic traders in this arena:
- Dynamic Regulations: Regulations can change often in response to market conditions or technological innovations. Staying updated is essential, and falling behind can be detrimental.
- Complex Reporting Requirements: Meeting compliance doesnât stop at knowing the rules; it also includes efficient documentation and reporting practices. Errors in reporting can lead to severe penalties.
- Data Security: Regulations often require robust measures to protect sensitive data. Implementing these security protocols can be both complicated and costly, especially for smaller trading firms.
- Cost of Compliance: The financial burden of compliance can be significant for firms, especially when investing in technology and hiring compliance professionals to ensure adherence to regulations.
Traders equipped with the right knowledge can navigate these obstacles effectively. After all, understanding compliance dynamics is not only about avoiding penalties; itâs also about seizing opportunities in a well-regulated trading environment, which ultimately can enhance trust and foster a healthier market.
"Knowing the rules of the game doesn't just keep you safe; it can give you the edge over competitors who ignore them."
The Future of Algorithmic Trading
In a world where technology continually reshapes how we engage with financial markets, the future of algorithmic trading holds paramount significance. As investment landscapes evolve, the integration of sophisticated algorithms isnât just a novelty; itâs become a cornerstone of modern trading strategies. For novice and seasoned investors alike, understanding where this technology is headed is crucial for navigating an increasingly complex financial terrain.
The confluence of advanced data analytics, artificial intelligence, and machine learning is setting the stage for transformative changes. These emerging trends not only promise enhanced performance but also pose new challenges that traders must reckon with. Adopting a forward-thinking approach to algorithmic trading can lead to substantial benefits, including improved speed in execution, better resource management, and the ability to react swiftly to market fluctuations.
Emerging Trends and Technologies
The rush of innovation in algorithmic trading is nothing short of exhilarating. Here are a few trends worth watching:
- Artificial Intelligence and Machine Learning: These technologies are evolving quickly, allowing algorithms to learn from historical data and adapt their strategies for better performance. Itâs akin to training a rookie investor by feeding him a mountain of past trades and their outcomes. Over time, the algorithm becomes more proficient at recognizing patterns.
- High-Frequency Trading (HFT): While HFT has been around for a while, the tools and methods behind it are advancing rapidly. Todayâs traders can capitalize on microsecond advantages, executing thousands of trades in the blink of an eye, thanks in large part to the rise of co-location solutions.
- Alternative Data Sources: Traditionally, traders relied heavily on economic indicators like GDP or unemployment rates. Now, information from social media, satellite imagery, and even web traffic analytics are becoming valuable inputs for trading strategies.
"In the future, the ability to leverage unconventional data could very well be what separates successful traders from the rest."
The technological realm is shifting. It is essential to stay informed on advancements in quantum computing as well. This could dramatically enhance the processing power available for complex algorithms, enabling traders to explore strategies previously deemed impractical.
Potential Impact on Retail Investors
As these trends unfold, the impact on retail investors cannot be overstated. Algorithmic trading has democratized access to sophisticated tools that were once exclusive to institutional investors.
- Accessibility of Advanced Tools: Most retail platforms now integrate algorithmic models, allowing individual traders to harness powerful strategies without needing in-depth programming knowledge. Platforms like Charles Schwab provide user-friendly interfaces that can adapt algorithmic principles into automated trade execution.
- Increased Competition: As retail investors gain access to same powerful tools, the playing field is leveling. This greater competition could potentially lead to tighter spreads and improved prices across various securities. However, it also means that investors need to be more astute than ever, as the margin for error shrinks.
- Enhanced Decision-Making: The insights gleaned from data analytics empower individual investors to make informed decisions faster. They can respond to market events in real-time, potentially capitalizing on opportunities that were missed in the past.
In summary, as algorithmic trading continues to evolve, it presents both opportunities and challenges for retail investors. Embracing these changes, while being aware of potential pitfalls, will be vital in staying ahead in this new era of trading.